Key Features
Eager search spaces
Automated search for optimal hyperparameters using Python conditionals, loops, and syntax
State-of-the-art algorithms
Efficiently search large spaces and prune unpromising trials for faster results
Easy parallelization

Parallelize hyperparameter searches over multiple threads or processes without modifying code
Code Examples
Optuna is framework agnostic. You can use it with any machine learning or deep learning framework.
A simple optimization problem:
- Define
objective
function to be optimized. Let's minimize(x - 2)^2
- Suggest hyperparameter values using
trial
object. Here, a float value ofx
is suggested from-10
to10
- Create a
study
object and invoke theoptimize
method over 100 trials
import optuna
def objective(trial):
x = trial.suggest_float('x', -10, 10)
return (x - 2) ** 2
study = optuna.create_study()
study.optimize(objective, n_trials=100)
study.best_params # E.g. {'x': 2.002108042}
You can optimize PyTorch hyperparameters, such as the number of layers and the number of hidden nodes in each layer, in three steps:
- Wrap model training with an
objective
function and return accuracy - Suggest hyperparameters using a
trial
object - Create a
study
object and execute the optimization
import torch
import optuna
# 1. Define an objective function to be maximized.
def objective(trial):
# 2. Suggest values of the hyperparameters using a trial object.
n_layers = trial.suggest_int('n_layers', 1, 3)
layers = []
in_features = 28 * 28
for i in range(n_layers):
out_features = trial.suggest_int(f'n_units_l{i}', 4, 128)
layers.append(torch.nn.Linear(in_features, out_features))
layers.append(torch.nn.ReLU())
in_features = out_features
layers.append(torch.nn.Linear(in_features, 10))
layers.append(torch.nn.LogSoftmax(dim=1))
model = torch.nn.Sequential(*layers).to(torch.device('cpu'))
...
return accuracy
# 3. Create a study object and optimize the objective function.
study = optuna.create_study(direction='maximize')
study.optimize(objective, n_trials=100)
See full example on Github
You can optimize Chainer hyperparameters, such as the number of layers and the number of hidden nodes in each layer, in three steps:
- Wrap model training with an
objective
function and return accuracy - Suggest hyperparameters using a
trial
object - Create a
study
object and execute the optimization
import chainer
import optuna
# 1. Define an objective function to be maximized.
def objective(trial):
# 2. Suggest values of the hyperparameters using a trial object.
n_layers = trial.suggest_int('n_layers', 1, 3)
layers = []
for i in range(n_layers):
n_units = trial.suggest_int(f'n_units_l{i}', 4, 128, log=True)
layers.append(L.Linear(None, n_units))
layers.append(F.relu)
layers.append(L.Linear(None, 10))
model = L.Classifier(chainer.Sequential(*layers))
...
return accuracy
# 3. Create a study object and optimize the objective function.
study = optuna.create_study(direction='maximize')
study.optimize(objective, n_trials=100)
See full example on Github
You can optimize TensorFlow hyperparameters, such as the number of layers and the number of hidden nodes in each layer, in three steps:
- Wrap model training with an
objective
function and return accuracy - Suggest hyperparameters using a
trial
object - Create a
study
object and execute the optimization
import tensorflow as tf
import optuna
# 1. Define an objective function to be maximized.
def objective(trial):
# 2. Suggest values of the hyperparameters using a trial object.
n_layers = trial.suggest_int('n_layers', 1, 3)
model = tf.keras.Sequential()
model.add(tf.keras.layers.Flatten())
for i in range(n_layers):
num_hidden = trial.suggest_int(f'n_units_l{i}', 4, 128, log=True)
model.add(tf.keras.layers.Dense(num_hidden, activation='relu'))
model.add(tf.keras.layers.Dense(CLASSES))
...
return accuracy
# 3. Create a study object and optimize the objective function.
study = optuna.create_study(direction='maximize')
study.optimize(objective, n_trials=100)
See full example on Github
You can optimize Keras hyperparameters, such as the number of filters and kernel size, in three steps:
- Wrap model training with an
objective
function and return accuracy - Suggest hyperparameters using a
trial
object - Create a
study
object and execute the optimization
import keras
import optuna
# 1. Define an objective function to be maximized.
def objective(trial):
model = Sequential()
# 2. Suggest values of the hyperparameters using a trial object.
model.add(
Conv2D(filters=trial.suggest_categorical('filters', [32, 64]),
kernel_size=trial.suggest_categorical('kernel_size', [3, 5]),
strides=trial.suggest_categorical('strides', [1, 2]),
activation=trial.suggest_categorical('activation', ['relu', 'linear']),
input_shape=input_shape))
model.add(Flatten())
model.add(Dense(CLASSES, activation='softmax'))
# We compile our model with a sampled learning rate.
lr = trial.suggest_float('lr', 1e-5, 1e-1, log=True)
model.compile(loss='sparse_categorical_crossentropy', optimizer=RMSprop(lr=lr), metrics=['accuracy'])
...
return accuracy
# 3. Create a study object and optimize the objective function.
study = optuna.create_study(direction='maximize')
study.optimize(objective, n_trials=100)
See full example on Github
You can optimize MXNet hyperparameters, such as the number of layers and the number of hidden nodes in each layer, in three steps:
- Wrap model training with an
objective
function and return accuracy - Suggest hyperparameters using a
trial
object - Create a
study
object and execute the optimization
import mxnet as mx
import optuna
# 1. Define an objective function to be maximized.
def objective(trial):
# 2. Suggest values of the hyperparameters using a trial object.
n_layers = trial.suggest_int('n_layers', 1, 3)
data = mx.symbol.Variable('data')
data = mx.sym.flatten(data=data)
for i in range(n_layers):
num_hidden = trial.suggest_int(f'n_units_l{i}', 4, 128, log=True)
data = mx.symbol.FullyConnected(data=data, num_hidden=num_hidden)
data = mx.symbol.Activation(data=data, act_type="relu")
data = mx.symbol.FullyConnected(data=data, num_hidden=10)
mlp = mx.symbol.SoftmaxOutput(data=data, name="softmax")
...
return accuracy
# 3. Create a study object and optimize the objective function.
study = optuna.create_study(direction='maximize')
study.optimize(objective, n_trials=100)
See full example on Github
You can optimize Scikit-Learn hyperparameters, such as the C
parameter of
SVC
and the max_depth
of the RandomForestClassifier
,
in
three steps:
- Wrap model training with an
objective
function and return accuracy - Suggest hyperparameters using a
trial
object - Create a
study
object and execute the optimization
import sklearn
import optuna
# 1. Define an objective function to be maximized.
def objective(trial):
# 2. Suggest values for the hyperparameters using a trial object.
classifier_name = trial.suggest_categorical('classifier', ['SVC', 'RandomForest'])
if classifier_name == 'SVC':
svc_c = trial.suggest_float('svc_c', 1e-10, 1e10, log=True)
classifier_obj = sklearn.svm.SVC(C=svc_c, gamma='auto')
else:
rf_max_depth = trial.suggest_int('rf_max_depth', 2, 32, log=True)
classifier_obj = sklearn.ensemble.RandomForestClassifier(max_depth=rf_max_depth, n_estimators=10)
...
return accuracy
# 3. Create a study object and optimize the objective function.
study = optuna.create_study(direction='maximize')
study.optimize(objective, n_trials=100)
See full example on Github
You can optimize XGBoost hyperparameters, such as the booster type and alpha, in three steps:
- Wrap model training with an
objective
function and return accuracy - Suggest hyperparameters using a
trial
object - Create a
study
object and execute the optimization
import xgboost as xgb
import optuna
# 1. Define an objective function to be maximized.
def objective(trial):
...
# 2. Suggest values of the hyperparameters using a trial object.
param = {
'silent': 1,
'objective': 'binary:logistic',
'booster': trial.suggest_categorical('booster', ['gbtree', 'gblinear', 'dart']),
'lambda': trial.suggest_float('lambda', 1e-8, 1.0, log=True),
'alpha': trial.suggest_float('alpha', 1e-8, 1.0, log=True)
}
bst = xgb.train(param, dtrain)
...
return accuracy
# 3. Create a study object and optimize the objective function.
study = optuna.create_study(direction='maximize')
study.optimize(objective, n_trials=100)
See full example on Github
You can optimize LightGBM hyperparameters, such as boosting type and the number of leaves, in three steps:
- Wrap model training with an
objective
function and return accuracy - Suggest hyperparameters using a
trial
object - Create a
study
object and execute the optimization
import lightgbm as lgb
import optuna
# 1. Define an objective function to be maximized.
def objective(trial):
...
# 2. Suggest values of the hyperparameters using a trial object.
param = {
'objective': 'binary',
'metric': 'binary_logloss',
'verbosity': -1,
'boosting_type': 'gbdt',
'lambda_l1': trial.suggest_float('lambda_l1', 1e-8, 10.0, log=True),
'lambda_l2': trial.suggest_float('lambda_l2', 1e-8, 10.0, log=True),
'num_leaves': trial.suggest_int('num_leaves', 2, 256),
'feature_fraction': trial.suggest_float('feature_fraction', 0.4, 1.0),
'bagging_fraction': trial.suggest_float('bagging_fraction', 0.4, 1.0),
'bagging_freq': trial.suggest_int('bagging_freq', 1, 7),
'min_child_samples': trial.suggest_int('min_child_samples', 5, 100),
}
gbm = lgb.train(param, dtrain)
...
return accuracy
# 3. Create a study object and optimize the objective function.
study = optuna.create_study(direction='maximize')
study.optimize(objective, n_trials=100)
See full example on Github
Check more examples including PyTorch Ignite, Dask-ML and MLFlow at our Github
repository.
It also provides the visualization demo as follows:
from optuna.visualization import plot_intermediate_values
...
plot_intermediate_values(study)
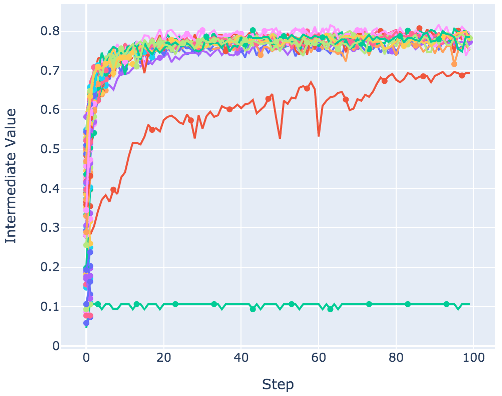
Installation
Dashboard
Optuna Dashboard is a real-time web dashboard for Optuna. You can check the optimization history, hyperparameter importances, etc. in graphs and tables.
% pip install optuna-dashboard
% optuna-dashboard sqlite:///db.sqlite3
Optuna Dashboard is also available as extensions for Jupyter Lab and Visual Studio Code.
VS Code Extension
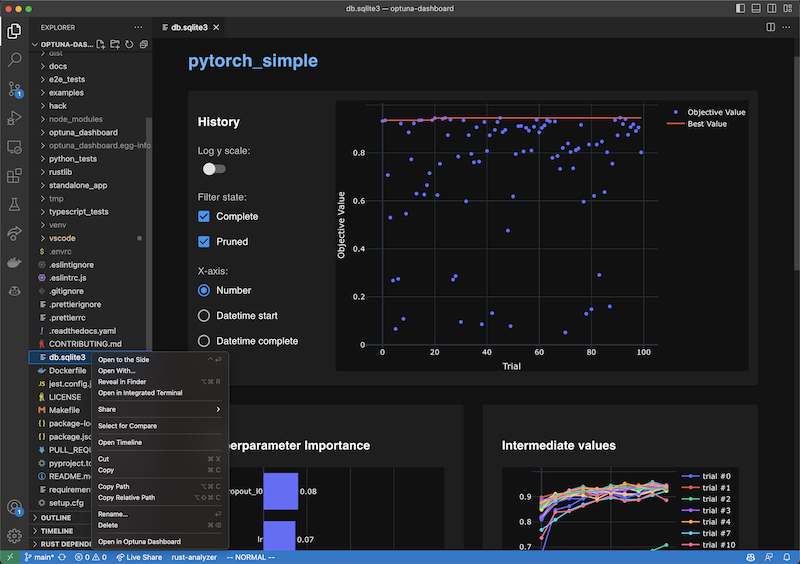
To use, install the extension, right-click the SQLite3 files in the file explorer and select the “Open in Optuna Dashboard” from the dropdown menu.
Jupyter Lab Extension
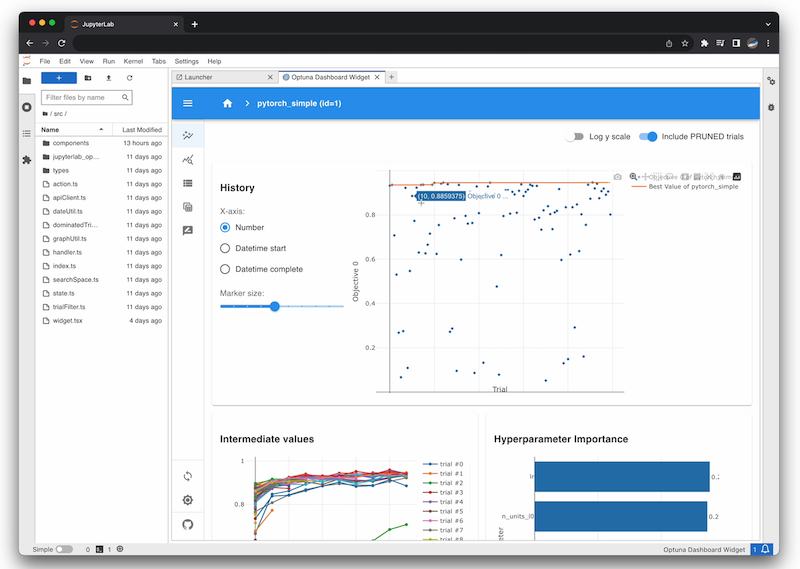
% pip install jupyterlab jupyterlab-optuna
OptunaHub
OptunaHub is a feature-sharing platform for Optuna. Users can freely use registered features, and contributors can register the features they implement.
% pip install optunahub
import optunahub
import optuna
def objective(trial):
x = trial.suggest_float("x", 0, 1)
return x
mod = optunahub.load_module("samplers/simulated_annealing")
sampler = mod.SimulatedAnnealingSampler()
study = optuna.create_study(sampler=sampler)
study.optimize(objective, n_trials=20)
Blog
Videos
Paper
If you use Optuna in a scientific publication, please use the following citation:
Takuya Akiba, Shotaro Sano, Toshihiko Yanase, Takeru Ohta, and Masanori Koyama. 2019. Optuna: A Next-generation Hyperparameter Optimization Framework. In KDD.View Paper arXiv Preprint
Bibtex entry:
@inproceedings{optuna_2019, title={Optuna: A Next-generation Hyperparameter Optimization Framework}, author={Akiba, Takuya and Sano, Shotaro and Yanase, Toshihiko and Ohta, Takeru and Koyama, Masanori}, booktitle={Proceedings of the 25th {ACM} {SIGKDD} International Conference on Knowledge Discovery and Data Mining}, year={2019} }